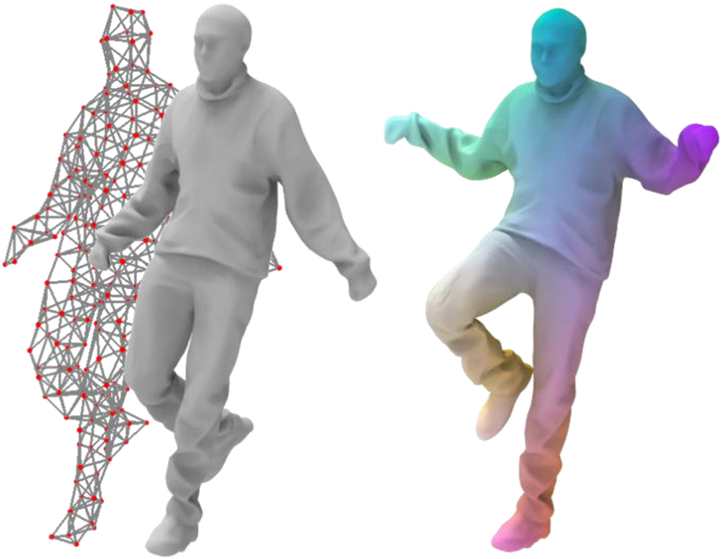 |
Differentiable Deformation Graph based Neural Non-rigid Registration
Wanquan Feng, Hongrui Cai,
Junhui Hou, Bailin Deng,
Juyong Zhang.
Communications in Mathematics and Statistics (CIMS), 2023
paper /
video
We propose a differentiable deformation graph based neural learning method for non-rigid registration by replacing some components with neural based strategies to fully take advantage of the shape priors and domain knowledge embedded in trained neural networks.
|
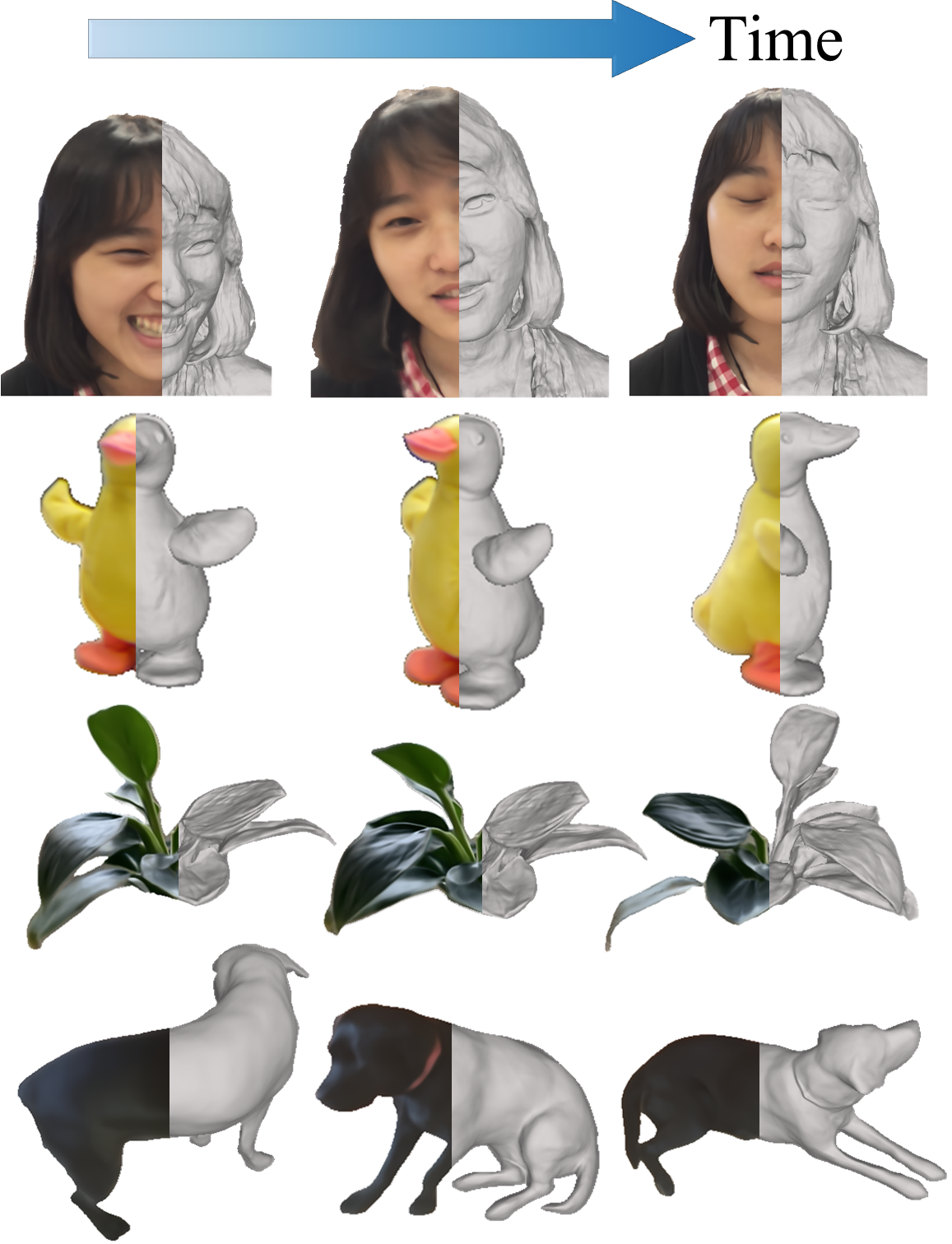 |
Neural Surface Reconstruction of Dynamic Scenes with Monocular RGB-D Camera
Hongrui Cai, Wanquan Feng,
Xuetao Feng, Yan Wang,
Juyong Zhang.
NeurIPS, 2022 (Spotlight)
project page /
paper /
OpenReview /
poster /
code
We propose Neural-DynamicReconstruction (NDR), a template-free method to recover high-fidelity geometry and motions of a dynamic scene from a monocular RGB-D camera. To represent and constrain the non-rigid deformations, we propose a novel neural invertible deforming network such that the cycle consistency between arbitrary two frames is automatically satisfied.
|
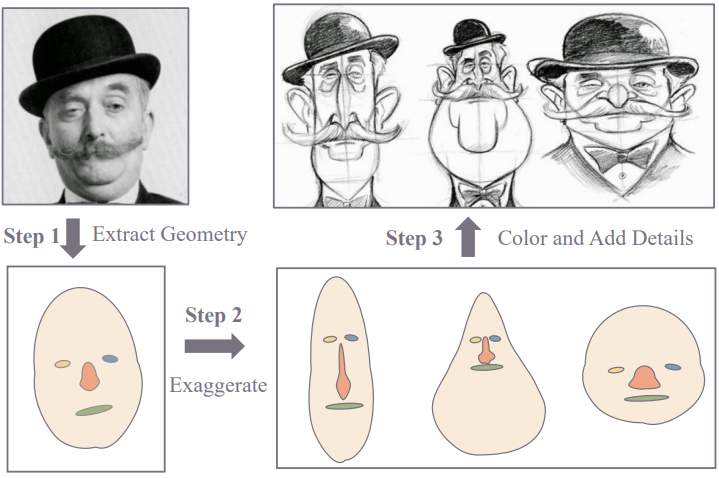 |
CariPainter: Sketch Guided Interactive Caricature Generation
Xin Huang, Dong Liang, Hongrui Cai,
Juyong Zhang, Jinyuan Jia.
ACM MM, 2022
paper & video
We propose CariPainter, the first interactive caricature generating and editing method, by utilizing the semantic segmentation maps as an intermediary domain.
|
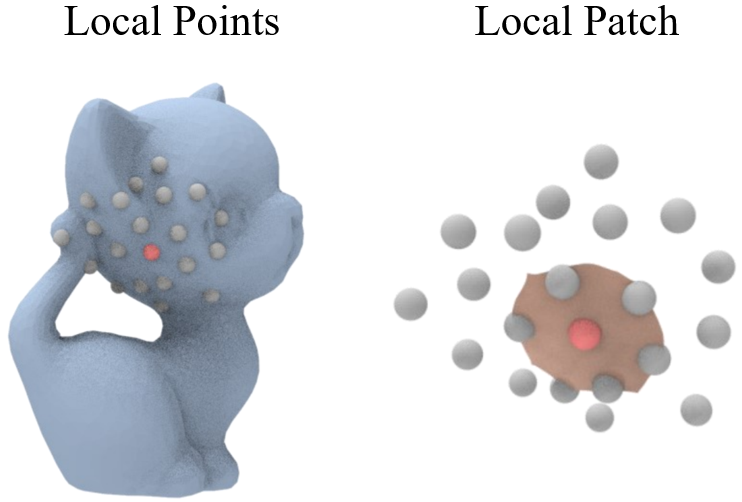 |
Neural Points: Point Cloud Representation with Neural Fields for Arbitrary Upsampling
Wanquan Feng, Jin Li,
Hongrui Cai, Xiaonan Luo,
Juyong Zhang.
CVPR, 2022
project page /
paper /
zhihu /
code
In this paper, we propose Neural Points, a novel point cloud representation. Different from traditional point cloud representation, each point in Neural Points represents a local continuous geometric shape via neural fields. Therefore, Neural Points can express more complex geometry shapes.
|
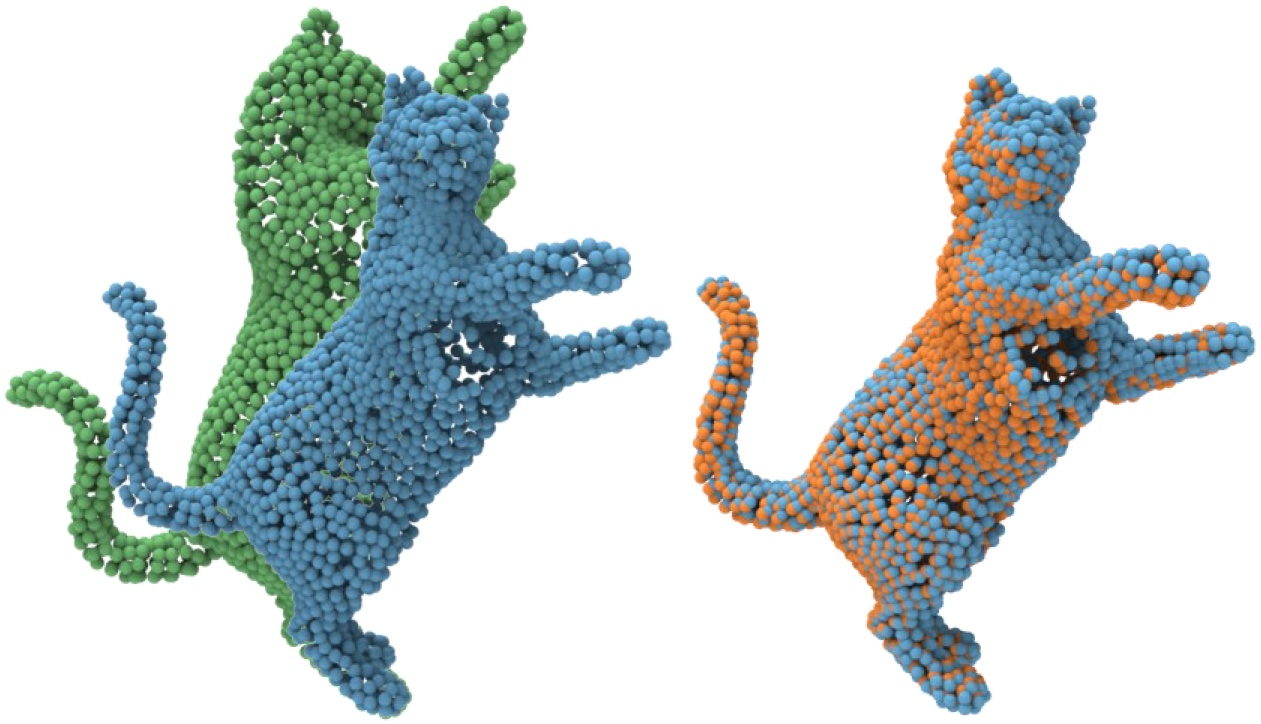 |
Recurrent Multi-view Alignment Network for Unsupervised Surface Registration
Wanquan Feng, Juyong Zhang,
Hongrui Cai, Haofei Xu,
Junhui Hou, Hujun Bao.
CVPR, 2021
project page /
paper /
zhihu /
code
For non-rigid registration, we propose RMA-Net to deform the input surface shape stage by stage. RMA-Net is trained in an unsupervised manner via our proposed multi-view 2D projection loss.
|